Parallel Computing & Algorithms
Goal
Multicore design is one of modern features of computer systems. You can easily see at least 4 cores in commercial CPUs in your desktop computers, laptops, even smart phones (for example, Apple A16 has one 6 cores CPU). However, the programming beginners rarely have opportunities to exploit the performance benefits of multi-core hardware, letting alone get a deep understanding of contemporary computer architecture. In recent years, Artificial Intelligence (AI) has become a prominent field, heavily relying on graphics processing units (GPUs) or accelerators for machine learning (ML). For example, the latest NVIDIA graphics card, the RTX 4090, has 16384 CUDA cores. Utilizing such an immense number of computational units has become a crucial challenge. This course shows you how to utilize these computing units (CPUs and GPUs) to unleash their computational performance. We will cover the following topics in ths course:
- Modern computer architecture and job scheduling in operating systems
- Multiprocessing & multithreading programming in Java, C#, and Python
- Parallel algorithms
- CUDA in C++
現今多核心設計的中央處理器 (central processing unit, CPU) 隨處可見 ,例如桌上型電腦、筆記型電腦甚至是智慧型手機,核心數至少 4 核心 (cores) 起跳,多可高達 96 核心 (例如 AMD EPYC 9654),但初學程式設計的學員鮮少有機會利用到硬體多核所帶來的效能,甚至還沒有機會深入了解當代的電腦架構。近年人工智慧 (Artificial Intelligence, AI) 成為顯學,大量需要繪圖晶片 (graphics processing unit, GPU) 或者圖形加速器來進行機器學習 (machine learning, ML),例如當前最新的 NVIDIA 顯示卡 RTX 4090 擁有 16384 顆 CUDA 核心,如何使用如此龐大的計算單元變成一個重要的課題。本課程將介紹平行程式與平行演算法,學員在課程中預計能夠學習到下列項目:
- 當代硬體架構、作業系統 (以 Linux 為例)
- 多程序與多執行緒的架構與實作 (以 Java、C#、Python 為例)
- 平行演算法
- CUDA (以 C++ 為例)
Textbook
TBA
Programming Languages
- You can pick up programming languages like C, C++, C#, Java, and Python mentioned in this course.
- You can find installation instructions here.
Syllabus
Background Knowledge
Type of Parallelism
- Type fo tasks
- CPU-bound tasks
- I/O-bound tasks
- For example, some operations of databases, web crawling.
- Parallelism in hardware
Image Not Showing
Possible Reasons
- The image file may be corrupted
- The server hosting the image is unavailable
- The image path is incorrect
- The image format is not supported
Learn More →
- Parallelism in a uniprocessor
- SIMD instructions, vector processors, GPUs
- Multiprocessors
- Symmetric shared-memory multiprocessors
- Distributed-memory multiprocessors
- Chip-multiprocessors a.k.a. multicores
- Multicomputers a.k.a. clusters
- Parallelism in software
- Instruction-level parallelism
- Task-level parallelism
- Data-level parallelism
- Transaction level parallelis
Preliminary Knowledge about Hardware
- Multitasking/programming computer
- Unlike mainframe computers which execute tasks in batches, personal computers (PC) need to perform multiple tasks simultaneously.
- Hardware acceleration
- Instruction pipelining: fetch, decode, execution, write-back
- Loop unrolling
- Out-of-order execution
- Register renaming
- Branch prediction
- Prefetching memory and files
- Cache design in modern processors
- Multi-core design
- https://www.techspot.com/article/2363-multi-core-cpu/
- In 2001 we saw the first true multi-core processor released by IBM under their Power4 architecture and as expected it was geared towards workstation and server applications.
- In 2005, however, Intel released its first consumer focused dual-core processor which was a multi-core design and later that same year AMD released their version with the Athlon X2 architecture.
- The first 1-qubit processors were announced not too long ago and yet a 54-Qubit processor was announced by Google in 2019 and claimed to have achieved quantum supremacy, which is a fancy way of saying their processor can do something a traditional CPU cannot do in a realistic amount of time.
- Series aritcles for computer hardware development are listed as follows: personal computer, cpu, memory, graphic card, mother board, hard drive, ssd
Preliminary Knowledge about Software: Operating System
-
Program vs. Process
Image Not Showing
Possible Reasons
- The image was uploaded to a note which you don't have access to
- The note which the image was originally uploaded to has been deleted
Learn More →
https://techdifferences.com/difference-between-program-and-process.html
-
Job scheduling
-
Thread
Image Not Showing
Possible Reasons
- The image file may be corrupted
- The server hosting the image is unavailable
- The image path is incorrect
- The image format is not supported
Learn More →
-
Parallelism and concurrency
- Write to database in parallel or concurrently?
- See atomicity of the ACID properties of database transactions.
-
Race condition
-
Deadlock
- Dining philosophers
Image Not Showing
Possible Reasons
- The image was uploaded to a note which you don't have access to
- The note which the image was originally uploaded to has been deleted
Learn More →
- Another issue: starvation
- How to prevent the deadlock?
- Try to eliminate the following four conditions:
- Mutual exclusion
- Non-preemption
- Hold and wait
- Circular wait
- Producer-consumer model
-
Amdhal's Law
- Let be the parallelizable portion of one algorithm in percentage and be the number of processors (or computing units of any type).
- For convenience, the timecost before parallelizing the algorithm is
- Then the timecost of the algorithm of parallel version is
- The Amdhal's law states that the asymptotic limit of the speedup is
- This implies that you cannot reduce the execution time to zero even if you can fully parallelize the algorithm.
- In conclusions,
- Parallelism benefits reduction of execution time until the marginal improvement starts to degrade as the non-parallelizable portion dominates gradually, one example for the law of diminishing returns.
- Instead of buying more machines, it's better to hire programmers who are good at parallel algorithms.
Image Not Showing
Possible Reasons
- The image was uploaded to a note which you don't have access to
- The note which the image was originally uploaded to has been deleted
Learn More →
Parallel Modules/Packages in Programming Languages
-
Linux
-
Python
- multiprocessing
- One of my daily jobs is to compute on intraday market data. For TXO (台指選擇權), the number of transaction entries is more than 20M on daily basis to calculate the TAIWAN VIX (台指選擇權波動率指數).
Image Not Showing
Possible Reasons
- The image was uploaded to a note which you don't have access to
- The note which the image was originally uploaded to has been deleted
Learn More →
- threading
- asyncio: asynchronous (nonblocking) programming
- References
-
Java sample code
- Basic level: the Thread class and the Runnable interface.
- Mid level: thread pool implemented by the Executors class and its associated members.
-
Goroutine
-
NVIDIA CUDA
-
C
- OpenMP
- POSIX Threads (aka pthread)
-
C++
- OpenMP
- Multithreaded programming
- More articles about C++ parallel programming
Parallel Algorithms
Practical Examples
Monte Carlo Simulation
- It is an example of embarrassingly (ideally) parallel algorithms.

need quantitative benchmark
Compression & Decompression
- Scenarios
- Case 1: Decompressing 266 zip files for the TX futures. The total size of all csv files is 5.6 GB.
- Case 2: Decompressing 254 zip files for all stocks in the Taiwan equity market. The total size of all csv files is 11 GB.
- Case 3: Compressing nnnn csv files for all stocks in the Taiwan equity market.
Scenario |
Sequential (1T) |
Parallel (48T) |
Case 1 |
36.915s |
1.539s |
Case 2 |
1m13.223s |
3.597s |
Case 3 |
TBA |
TBA |
Web Crawling
need quantitative benchmark
Complexity Analysis
- Can parallism reduce the time complexity of one problem?
- Definitely impossible unless you can supply processors/workers as many as the number of data size with a constant ratio.
Distributed Computing
- Message Passing Interface (MPI)
References
Books
- W. P. Petersen and P. Arbenz, Introduction to Parallel Computing, 2004

- Peter Pacheco and Matthew Malensek, An Introduction to Parallel Programming, 2/e, 2022

- Roman Trobec, Boštjan Slivnik, Patricio Bulić, and Borut Robič, Introduction to Parallel Computing: From Algorithms to Programming on State-of-the-Art Platforms, 2018

Operating Systems (OS)
- Abraham Silberschatz, Greg Gagne, Peter B. Galvin, Operating System Concepts, 10/e, 2021

- Andrew S. Tanenbaum and Herbert Bos, Modern Operating Systems, 4/e, 2015

- Remzi H. Arpaci-Dusseau and Andrea C. Arpaci-Dusseau, Operating Systems: Three Easy Pieces, 2018

Hardware Architectures
- Ulrich Drepper, What Every Programmer Should Know About Memory, 2007
- Maurice Herlihy, Nir Shavit, Victor Luchangco, and Michael Spear, The Art of Multiprocessor Programming, 2/e, 2020
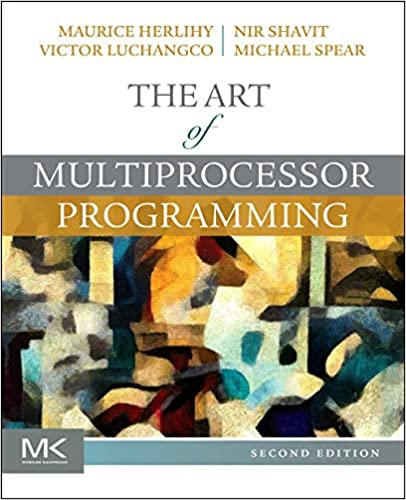
- Randal Bryant and David O'Hallaron, Computer Systems: A Programmer's Perspective, 3/e, 2015

Parallel Algorithms
- Behrooz Parhami, Introduction to Parallel Processing: Algorithms and Architectures, 1999

- Frank Thomson Leighton, Introduction to Parallel Algorithms and Architectures: Arrays, Trees, Hypercubes, 1991

- Michael McCool, James Reinders, and Arch Robison, Structured Parallel Programming: Patterns for Efficient Computation, 2012

CUDA
Distributed Computing
Courses
- Aydin Buluc and Jim Demmel, Applications of Parallel Computers, UC Berkeley, 2022
- Suresh Jagannathan, CS 353: Principles of Concurrency and Parallelism, Purdue University, 2022sp
- Brian Railing and Nathan Beckmann, 15-418/15-618: Parallel Computer Architecture and Programming, Carnegie Mellon University, 2021sp
- High Performance Computing For Science And Engineering (HPCSE) II, Swiss Federal Institute of Technology in Zurich
- Bei Yu, CMSC 5743 Efficient Computing of Deep Neural Networks, The Chinese University of Hong Kong, 2021
- Dan Garcia and Lisa Yan, Great Ideas in Computer Architecture (Machine Structures), UC Berkeley, 2022fa
- Yonghong Yan, CSCE 513 Computer Architecture, University of South Carolina, 2018fa
- Mike Giles and Wes Armour, Course on CUDA Programming on NVIDIA GPUs, 2023
- Sergio Martin, High Performance Computing for Science and Engineering, 2021: pdf
- Al Barr, GPU Programming, Caltech, 2022
- Jserv, Linux 核心設計: 記憶體管理
Youtubes
Game
TODO
-
Case study
- Producer-consumer model
- Parallelism of Fibonacci numbers
- Single Instruction Multiple Data (SIMD)
-
Supplementary materials