# software pipelining
contributed by <`yangyang95`> <`changyuanhua`>
Github: [prefetcher](https://github.com/yangyang95/prefetcher)
錄影: ==[software-pipelining 報告](https://youtu.be/DJb7d_artUg)==
## 開發環境
* 輸入指令 ` lscpu `
```
Architecture: x86_64
CPU 作業模式: 32-bit, 64-bit
Byte Order: Little Endian
CPU(s): 4
On-line CPU(s) list: 0-3
每核心執行緒數:1
每通訊端核心數:4
Socket(s): 1
NUMA 節點: 1
供應商識別號: GenuineIntel
CPU 家族: 6
型號: 94
Model name: Intel(R) Core(TM) i5-6300HQ CPU @ 2.30GHz
製程: 3
CPU MHz: 799.890
CPU max MHz: 3200.0000
CPU min MHz: 800.0000
BogoMIPS: 4608.00
虛擬: VT-x
L1d 快取: 32K
L1i 快取: 32K
L2 快取: 256K
L3 快取: 6144K
NUMA node0 CPU(s): 0-3
```
## 預期目標
- [ ] 彙整 [2017q1 homework3](https://hackmd.io/s/HkYlSCJil) 的開發成果
- [x]prefetch distance
- [x]locality hint
- [ ]Memory latency check
- [x]perf raw counter
- [x]execute experiment for many times and make plot
- [x]物件導向封裝
- [x]change array size
- [x]change _mm_prefetch 指令位置
- [x]stopwatch
- [ ] 針對 Cache
- [x] Cache 相關知識整理
* Cache 基本認識
* Cache latency
* Cache line
* Cache 的設計模式
* Cache miss
- [ ] 實驗結果分析,並完整解釋原因
- [ ] 記錄並回答老師課堂上的提問
## 問題紀錄
1. prefetch 指令是否會佔 CPU cycle?
ANS: 會
### CPU Cycle 運作
處理一道處理器指令 (instruction) 時,資料會傳送或來自記憶體或輸入/輸出單元。如此每一次的傳送或接收的動作稱為機器週期(Machine Cycle)
機器週期又可分為兩部份:
* 指令週期 (Instruction cycle,I-cycle)
* 執行週期(Execution cycle,E-cycle)
**指令週期**
1. 控制單元從主記憶體中提取下一個所要執行的指令
2. 控制單元從指令予以解碼(decode)
3. 控制單元將指令中用來說明要執行什麼動作的這一部份,存入指令暫存器
4. 控制單元將指令中用來說明相關資料所儲存之位置這一部份,存入位址暫存器
**執行週期**
1. 控制單元根據位址暫存器內的資訊,從主記憶體中讀啟所需的資料,並將其存入ALU的儲存暫存器內
2. 控制單元根據指令暫存的資訊,命令ALU去執行所需的運算
3. ALU執行所需之運算,它會將發現於儲存暫存器以及累加器內的數值予以相加
4. 運算結果再存回累加器內,這個動作會清除累加器原先所儲存的數值

reference:
* [CPU 結構與工作原理](https://market.cloud.edu.tw/content/senior/computer/ks_ks/et/cpu/index.htm)
2. pipeline stall? 如何克服?
ANS: "pipeline stall" is the delay caused on a processor using pipelines when a transfer of control is taken. Normally when a control-transfer instruction (a branch, conditional branch, call or trap) is taken, any following instructions which have been loaded into the processor's pipeline must be discarded or "flushed" and new instructions loaded from the branch destination. This introduces a delay before the processor can resume execution.
簡單來說,pipeline stall 是控制轉移指令(controll-transfer instruction)產生時,已被讀取到處理器 pipeline 的指令就必須被迫拋棄或被沖刷(flushed),這個過程所造成的延遲。
* refernce: http://www.mondofacto.com/facts/dictionary?pipeline+stall
## Cache 介紹
### Cache 基本認識
CPU cache 是用於減少處理器存取記憶體所需平均時間的機制,下圖為處理器架構:
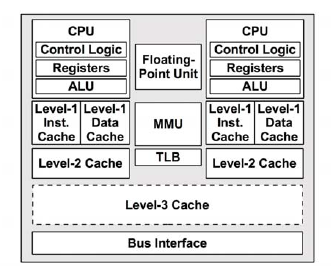
>註:圖中的 l2 cache 沒有跟其他 cpu 共享,l3 cache 有共享
* reference : [photo](http://zhengheng.me/content/images/2015/11/perf_3.png)
1. level-1 data cache: 一級資料快取(D$)
2. level-1 inst cache: 一級指令快取(I$)
3. MMU:記憶體管理單元
4. TLB:translation lookaside buffer
5. level-2 cache: 二級快取
6. level-3 cache: 三級快取
處理器讀取資料的路徑如下圖:
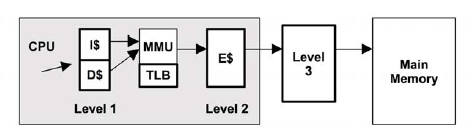
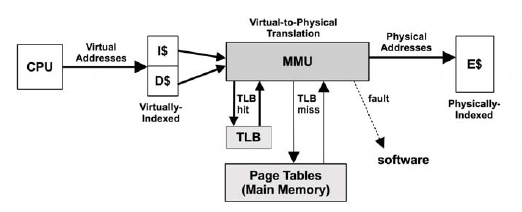
整個過程大致如下:
1. CPU 根據虛擬地址嘗試從一級快取 (存放的是虛擬地址的索引) 中讀取資料;
2. 如果一級快取中查找不到,則需向 MMU 請求資料;
3. MMU 從 TLB 中查找虛擬地址的快取 (換言之,TLB 是負責改進虛擬地址到實體地址轉換速度、存放虛擬地址的快取);
4. 若 TLB 中存在該虛擬地址的快取,則 MMU 將該虛擬地址轉化為實體地址,如果地址轉換失敗,則發生缺頁 (圖中的 fault 分支),由作業系統核心進行處理,見上文所述;如果地址轉換成功,則從二級快取 (存放的是實體地址的索引) 中讀取;如果二級快取中也沒有,則需要從三級快取,甚至實體記憶體中請求;
5. 如果 TLB 中不存在該虛擬地址的快取,則 MMU 從實體記憶體中的轉換表 (translation tables,也稱為分頁表 page tables) 中獲取,同時存入 TLB;(注意,這個操作由硬體實作,可由 MMU 通過硬體直接從實體記憶體中讀取);
6. 跳到第4步。
reference:
* [perf stat 輸出解讀](http://zhengheng.me/2015/11/12/perf-stat/)
### Cache latency
在一般的電腦上,指令大概的執行時間(相對):
| execute typical instruction | 1/1,000,000,000 sec = 1 nanosec |
|-------------------------------------|----------------------------------------|
| fetch from L1 cache memory | 0.5 nanosec |
| branch misprediction | 5 nanosec |
| fetch from L2 cache memory | 7 nanosec |
| Mutex lock/unlock | 25 nanosec |
| fetch from main memory | 100 nanosec |
| send 2K bytes over 1Gbps network | 20,000 nanosec |
| read 1MB sequentially from memory | 250,000 nanosec |
| fetch from new disk location (seek) | 8,000,000 nanosec |
| read 1MB sequentially from disk | 20,000,000 nanosec |
| send packet US to Europe and back | 150 milliseconds = 150,000,000 nanosec |
reference:
* [Teach Yourself Programming in Ten Years](http://norvig.com/21-days.html#answers)
### Cache line
* cache line 是 cpu cache 中的最小快取單位。
* 主流的 CPU Cache 的 Cache Line 大小多為 64 Bytes
* 有些例外,如 ARMv8-A 為基礎的 Cavium ThunderX 的 cache line 為 128 Bytes
* Reference: [Cavium ThunderX](http://www.7-cpu.com/cpu/ThunderX.html)
* 假設有一個 512 bytes 的一級快取,那按照 64 Bytes 的快取單位大小來算,這個一级快取所能存放的快取個數就是 512/64 = 8 個。
```
cache line size 為 64 bytes
L1D size 為 512 bytes
cache line #0 " 64 bytes "
cache line #1 " 64 bytes "
cache line #2 " 64 bytes "
cache line #3 " 64 bytes "
cache line #4 " 64 bytes "
cache line #5 " 64 bytes "
cache line #6 " 64 bytes "
cache line #7 " 64 bytes "
```
### Cache 的設計模式
快取記憶體有三種對映函數 (mapping function):
* Direct Mapped
* Fully Associative
* n-way associative

#### 1. Direct Mapped
一塊資料(佔一個 cache line 的空間),只有一個 cache line 與之對應。為多對一的映射關係。在 1990 年代初期,直接映射是當時最流行的機制,因為所需的硬體資源非常有限。現在直接映射機制正在逐漸被淘汰。
**下面用停車場當作例子來做說明**:
假設有 1000 個停車位,每個位子依 000~999 的順序給一個號碼,你的學生證號碼的前三碼就是你的停車位號碼。這就是 cache 對映最簡單的例子。
然而這會有什麼問題呢?數字小的號碼(如:200多)可能有更大的機會同一個位置有多個學生要停。數字大的號碼就可能會比較空,因為學生證號碼900多開頭的學生可能不多。
**優點**:
* 簡單,記憶體中的每一個位置只會對應到 cache 中的一個特定位置。
**缺點**:
* 兩個變數映射到同一個 cache line 時,他們會不停地把對方替換出去。由於嚴重的衝突,頻繁刷新 cache 將造成大量的延遲,而且在這種機制下,如果沒有足夠大的 cache,處理程序幾乎無時間局部性可言。
#### 2. Fully Associative
在一個 Cache 集內,任何一個記憶體地址的數據可以被 cache 在任何一個 Cache Line 裡。
**下面用停車場當作例子來做說明**:
停車許可證比停車位還要多的狀況。有 1000 個停車位,但是有 5000 個同學。
**優點**:
* 可以完整利用記憶體
**缺點**:
* cache 中尋找 block 時需要搜尋整個 cache
#### 3. N-way Set Associative Cache
直接映射會遭遇衝突的問題,當多個塊同時競爭 cache 的同一個 cache line 時,它們不停地將對方踢出快取,這將降低命中率。
另一方面,完全關聯過於複雜,很難在硬體層面實現。
N路關聯快映射則是直接映射跟全映射的混合,在兩者之前取得平衡。
**下面用停車場當作例子來做說明**:
假設有 1000 個停車位,分成 10 組,使用 00~99 作為每一組內車位的編號。你的停車位就是學生證的前兩碼,如此一來你就有 10 個停車位可以選擇。
**優點**:
* 給定一個記憶體地址可以唯一對應一個 set ,對於 set 中只需遍歷 16 個元素就可以確定對象是否在快取中。
* 相對 Direct Mapped,連續熱點數據 (會導致一個 set 內的 conflict) 的區間更大
reference:
* [Direct Mapped Cache](https://www.cs.umd.edu/class/sum2003/cmsc311/Notes/Memory/direct.html), [Fully Associative Cache](https://www.cs.umd.edu/class/sum2003/cmsc311/Notes/Memory/fully.html), [Set Associative Cache](https://www.cs.umd.edu/class/sum2003/cmsc311/Notes/Memory/set.html)
* [關於 CPU Cache:程序猿需要知道的那些事](https://read01.com/0A6Dxg.html)
* [Cache基本原理之:結構](http://www.jianshu.com/p/2b51b981fcaf)
### Cache miss
**cache miss** 有三種:
* **Compulsory misses(強迫性失誤)**:也稱為 cold start misses,第一次存取未曾在 cache 內的 block 而發生的 cache miss ,這種 miss 是不可避免的。
* **Capacity misses(空間性失誤)**:因為在程式執行期間, cache 無法包含所有需要的 block 而產生的 cache miss。發生在一個 block 被取代後,稍後卻又需要用到。
* **Conflict misses(衝突性失誤)**:發生在 set-associative 或 direct-mapped caches ,當多個 blocks 競爭相同的 set。通常也稱作 collision misses。
**比較 capacity misses 和 conflict miss 的差異**
* 假設有 32KB 的 cache
* **情況一(capacity misses)**:如果有一個 64KB 的陣列需要重複存取,因為數組大小遠大於 cache 大小,所以沒辦法全部放入 cache 。第一次存取陣列發生的 cache misses 全都是 compulsory misses 。但之後存取陣列發生的 cache misses 全都是 capacity misses ,這時的 cache 已存滿,容量不足以儲存全部的資料。
* **情況二(conflict misses)**:如果有兩個 8KB 的數組需要來回存取,但是這兩個數組都映射到相同的地址, cache 的大小足夠儲存全部的數據,但是因為相同地址發生衝突,需要來回替換,這時候發生的 cache misses 全都是 conflict misses(不過,第一次存取陣列發生的 cache misses 仍然都是 compulsory misses),這時 cache 並沒有存滿。
**發生 conflict misses 的替換,有三種方法:**
* **LRU(least recently used,最近罕用)**
* 將集合中目前最不常用的的區塊加以取代
* 可減少失誤次數,實作困難且成本高,常用在虛擬記憶體中
e.g. 設block frame=4,進入的block順序為1,3,2,5,6,7,2,4,5,2,5,3,1,2,則2,2,5,2被hit
| 1 |3 |2 |5 |6 |7 |2 |4 |5 |2 |5 |3 |1 |2|
|:----:|:------:|:-----------:|:------:|:-----------:|:------:|:-----------:|:------:|:-----------:|:------:|:-----------:|:------:|:-----------:|:------:|:-----------:|------:|:-----------:|:------:|:-----------:|
| | | |1| 3 |2| 5 |6 |7 |7| 7| 4| 2| 5|
| | |1 |3 |2| 5| 6| 7 |2| 4| 4| 2| 5| 3|
| |1 |3 |2 |5| 6| 7| 2 |4| 5| 2| 5| 3| 1|
|1 |3 |2 |5 |6| 7| 2| 4 |5| 2| 5| 3| 1| 2|
* **FIFO(first in first out,先進先出)**
* 將集合中最早進入的區塊加以取代
* 實作較簡單,失誤較高
e.g. 設block frame=4,進入的block順序為1,3,2,5,6,7,2,4,5,2,5,3,1,2,則2,5,2被hit
| 1 |3 |2 |5 |6 |7 |2 |4 |5 |2 |5 |3 |1 |2|
|:----:|:------:|:-----------:|:------:|:-----------:|:------:|:-----------:|:------:|:-----------:|:------:|:-----------:|:------:|:-----------:|:------:|:-----------:|------:|:-----------:|:------:|:-----------:|
| | | |1| 3 |2| 2 |5 |5 |6| 7| 4| 2| 2|
| | |1 |3 |2| 5| 5| 6 |6| 7| 4| 2| 5| 5|
| |1 |3 |2 |5| 6| 6| 7 |7| 4| 2| 5| 3| 3|
|1 |3 |2 |5 |6| 7| 7| 4 |4| 2| 5| 3| 1| 1|
* **random(隨機選取)**
* 由集合中隨機選一個區塊加以取代
* 實作最簡單,常用在快取記憶體
* reference: [替代方法](http://systw.net/note/af/sblog/more.php?id=252)
## 重複執行實驗並且作圖分析
* 參考 [0xff07 HackMD 筆記](https://hackmd.io/s/H1d_W-gsg)
* 在 makefile 檔中,加入下列程式碼 -> 多次執行實驗
```shell
for i in `seq 1 100`; do \
printf "$$i " >> time.txt ; \
./naive_transpose >> time.txt ; \
printf " " >> time.txt; \
./sse_transpose >> time.txt; \
printf " " >> time.txt; \
./sse_prefetch_transpose >> time.txt; \
printf "\n" >> time.txt ; \
done
```
## 改變 `_mm_prefetch` 指令位置
* prefetch 指令的主要目的,是提前讓 CPU 載入稍後運算所需要的資料。
* 通常是在對目前的資料進行運算之前,告訴 CPU 載入下一筆資料。
* 這樣就可以讓目前的運算,和載入下一筆資料的動作,可以同時進行。
> ==Q:== prefetch 能不能預取 指令 cache 和 data cache

* reference: [photo](https://zh.wikipedia.org/wiki/%E5%93%88%E4%BD%9B%E7%BB%93%E6%9E%84#/media/File:Harvard_architecture.svg)
* **Harvard architecture**
此種架構,分別儲存 instructions 和 data
單獨存儲表示程式和資料儲存可分處不同地方。也表示著指令預取得以與其他活動並行。
* reference:[Harvard architecture](https://en.wikipedia.org/wiki/Harvard_architecture)

* reference: [photo](https://upload.wikimedia.org/wikipedia/commons/e/e5/Von_Neumann_Architecture.svg)
* **von Neumann architecture**
簡單來說,此種架構部分的處理單元中含有
1.一個算術邏輯單元 (arithmetic logic unit) 和處理器暫存器 (processor registers)
2.含有指令暫存器和程式計數器的控制單元
3.含有一個儲存單元來保存資料和指令
4.輸入和輸出機制
此種架構的指令獲取和數據操作不能同時發生,因為它們共享公共匯流排。這被稱為 von Neumann 瓶頸,並經常限制系統的性能
* reference:[Von Neumann architecture](https://en.wikipedia.org/wiki/Von_Neumann_architecture)
### sse prefetch
```
sse prefetch 1 沒有改變_mm_prefetch 指令位置
sse prefetch 2 改變_mm_prefetch 指令位置
```

執行 100 次後,平均時間分別為
```
sse prefetch 1: 56088.767
sse prefetch 2: 55974.668
```
時間為原本的 99.797%,減少的並不是很明顯,僅約 0.2% 的差距
* perf
* 沒有改變 _mm_prefetch 指令位置
```
Performance counter stats for './sse_prefetch_transpose' (50 runs):
10,839,523 cache-misses # 60.111 % of all cache refs ( +- 0.33% )
18,032,645 cache-references ( +- 0.24% )
1,813,591,769 instructions # 2.65 insn per cycle ( +- 0.00% )
683,678,792 cycles ( +- 0.08% )
0.220839448 seconds time elapsed ( +- 0.31% )
```
* 改變 _mm_prefetch 指令位置
```
Performance counter stats for './sse_prefetch_transpose' (50 runs):
11,172,775 cache-misses # 60.703 % of all cache refs ( +- 0.26% )
18,405,763 cache-references ( +- 0.20% )
1,813,547,154 instructions # 2.71 insn per cycle ( +- 0.00% )
668,991,058 cycles ( +- 0.14% )
0.214681570 seconds time elapsed ( +- 0.39% )
```
兩個 cache misses 的值並沒有相差多少
* perf raw counter:
* r014c: LOAD_HIT_PRE.SW_PF
* r024c: LOAD_HIT_PRE.HW_PF
``` perf stat -e r014c ./sse_prefetch_transpose ```
* 沒有改變 _mm_prefetch 指令位置
SW hit: 286700
HW hit: 253292
``` perf stat -e r024c ./sse_prefetch_transpose ```
* 改變 _mm_prefetch 指令位置
SW hit: 1537262
HW hit: 259003
### avx prefetch
```
avx prefetch 1 改變_mm_prefetch 指令位置
avx prefetch 2 沒有改變_mm_prefetch 指令位置
```

執行 100 次後,平均時間分別為
```
avx prefetch 1: 47355.499
avx prefetch 2: 50230.853
```
>==Q:== 討論為什麼圖片會這樣
時間為原本的 94.276 %,大約減少 6 %
* perf
* 改變 _mm_prefetch 指令位置
```
Performance counter stats for './avx_prefetch_transpose' (50 runs):
14,018,457 cache-misses # 62.922 % of all cache refs ( +- 0.15% )
22,279,089 cache-references ( +- 0.13% )
1,684,193,250 instructions # 2.50 insn per cycle ( +- 0.00% )
672,647,334 cycles ( +- 0.16% )
0.225615808 seconds time elapsed ( +- 0.40% )
```
* 沒有改變 _mm_prefetch 指令位置
```
Performance counter stats for './avx_prefetch_transpose' (50 runs):
13,336,332 cache-misses # 58.380 % of all cache refs ( +- 0.35% )
22,843,932 cache-references ( +- 0.23% )
1,684,019,663 instructions # 2.49 insn per cycle ( +- 0.00% )
676,128,022 cycles ( +- 0.07% )
0.224623318 seconds time elapsed ( +- 0.25% )
```
改變 _mm_prefetch 指令位置後, cache misses 反而增加,由原本 58.380 % 增至 62.922 % ,大約增加 4.5%
* perf raw counter:
* r014c: LOAD_HIT_PRE.SW_PF
* r024c: LOAD_HIT_PRE.HW_PF
``` perf stat -e r014c ./avx_prefetch_transpose ```
* 沒有改變 _mm_prefetch 指令位置
SW hit: 2030
HW hit: 259499
``` perf stat -e r024c ./avx_prefetch_transpose ```
* 改變 _mm_prefetch 指令位置
SW hit: 3900
HW hit: 268269
## perf raw counter
* 參考 [linux perf example](http://www.brendangregg.com/perf.html)
其中說明在有些系統架構中,大多數的 counter 並不能被 perf list 找到,但如果你找到一個你想用的 counter ,你可以將它指定為一個 raw event (格式:rUUEE,UU == umask,EE == event)
* example:
```
# perf stat -e cycles,instructions,r80a2,r2b1 gzip file1
Performance counter stats for 'gzip file1':
5,586,963,328 cycles # 0.000 GHz
8,608,237,932 instructions # 1.54 insns per cycle
9,448,159 raw 0x80a2
11,855,777,803 raw 0x2b1
1.588618969 seconds time elapsed
```
`r80a2`,其中`80`是 mask number,`a2` 是 event number,可以查出 `raw 0x80a2` 是 RESOURCE_STALLS.OTHER 用來查看因為其它 resource issues 而被 stall 的 cycle數。`r2b1`同理是`UOPS_DISPATCHED.CORE`
* perf raw counter:
* r014c: LOAD_HIT_PRE.SW_PF
* r024c: LOAD_HIT_PRE.HW_PF
* r02D1: L2 cache hit
* r10D1: L2 cache miss
* r04D1: L3 cache hit
* r20D1: L3 cache miss
* 參考[kaizsv HackMD 筆記](https://hackmd.io/s/r1IWtb9R#sse-prefetch-transpose)
在 [Intel® 64 and IA-32 Architectures Developer's Manual: Vol. 3B](http://www.intel.com/content/www/us/en/architecture-and-technology/64-ia-32-architectures-software-developer-vol-3b-part-2-manual.html) 的19章,可以從自己的 CPU 型號查到對應的 mask number 及 event number
**raw counter 測試數據參考**
```clike=
Performance counter stats for './sse_prefetch_transpose' (10 runs):
753,555,879 L1-dcache-loads ( +- 1.41% ) (20.78%)
12,533,750 L1-dcache-load-misses 1.66% of all L1-dcache hits ( +- 4.66% ) (19.03%)
298,091,414 L1-dcache-stores ( +- 1.65% ) (11.40%)
3,626,299 L1-dcache-store-misses ( +- 5.59% ) (12.06%)
/* <not supported> L1-dcache-prefetches */
1,174,323 L1-dcache-prefetch-misses ( +- 8.22% ) (11.92%)
/* <not supported> L1-icache-loads */
127,860 L1-icache-load-misses ( +- 17.28% ) (18.31%)
/* <not supported> L1-icache-prefetches */
/* <not supported> L1-icache-prefetch-misses */
3,418,508 LLC-loads ( +- 6.44% ) (24.06%)
/* <not supported> LLC-load-misses */
3,219,088 LLC-stores ( +- 2.30% ) (23.69%)
/* <not supported> LLC-store-misses */
997,209 LLC-prefetches ( +- 12.67% ) (11.63%)
/* <not supported> LLC-prefetch-misses */
675,085,070 dTLB-loads ( +- 1.68% ) (11.70%)
4,973,121 dTLB-load-misses # 0.74% of all dTLB cache hits ( +- 9.00% ) (11.57%)
270,220,422 dTLB-stores ( +- 0.92% ) (11.41%)
163,715 dTLB-store-misses ( +- 3.27% ) (11.19%)
/* <not supported> dTLB-prefetches */
/* <not supported> dTLB-prefetch-misses */
2,221 iTLB-loads ( +- 15.88% ) (10.96%)
3,428 iTLB-load-misses # 154.37% of all iTLB cache hits ( +- 38.32% ) (16.47%)
261,610,532 branch-loads ( +- 3.23% ) (21.68%)
509,599 branch-load-misses ( +- 3.42% ) (21.35%)
1,994,238 r014c /* LOAD_HIT_PRE.SW_PF */ ( +- 12.91% ) (21.05%)
486,587 r024c /* LOAD_HIT_PRE.HW_PF */ ( +- 23.72% ) (20.91%)
0.376223174 seconds time elapsed ( +- 1.29% )
```
## locality hint
* **prefetch 的意義**
如果在CPU操作數據之前,我們就已經將數據提前加載到快取中,那麼就減少了由於快取未中 (cache-miss),需要從記憶體取值的情況,這樣就可以加速操作,獲得性能上提升。使用主動快取技術來優化記憶體複製。
值得注意的是,CPU 對數據操作擁有絕對自由!使用預取指令只是按我們自己的想法對 CPU 的數據操作進行補充,有可能CPU當前並不需要我們加載到快取的數據。這樣,我們的預取指令可能會帶來相反的結果,比如對於多工系統,有可能我們沖掉了有用的 cache。不過,在多工系統上,由於執行緒 (thread) 或行程 (process) 的切換所花費的時間相對於預取操作來說太長了, 所以可以忽略執行緒或行程切換對預取的影響。
> ==Q:== locality
locality of reference(存取的局部性)
說明: 大多數的存取都集中在極少數的指令或資料上,其他多數的指令或資料則很少被取用
幫助: 讓快取記憶體只需很小的空間就可存放這些少數重覆的指令或資料,提高擊中率使平均存取時間接近快取記憶體時間,提高效能
可分為:
* temporal locality(時間的局部性): 一個記憶體位址被存取後,不久會再度被存取
* 如: 迴圈,副程式,以及堆疊,迴圈控制變數,計算總合變數
* spatial locality (空間的局部性): 一個記憶體位址被存取後,不久其附近的記憶體位址也會被存取
* 如: 循序指令、陣列,和相關的變數
ps:無存取局部性的有分支指令、雜湊搜尋表、二元搜尋...等
* reference: [locality of reference](http://systw.net/note/af/sblog/more.php?id=135)
> ==Q:== cache invalidate
Cache invalidation is a process in a computer system whereby entries in a cache are replaced or removed. It can be done explicitly, as part of a cache coherence protocol. In such a case, a processor changes a memory location and then invalidates the cached values of that memory location across the rest of the computer system.
* reference: [Cache invalidation](https://en.wikipedia.org/wiki/Cache_invalidation)
> ==Q:== cache invalidate VS cache flush
–Flush
把 Cache 內容寫回 Memory ,當 Cache 為 Write through ,不需要 Flush
–Invalidate
把 Cache 內容直接丟掉不要
* reference: [cache invalidate VS cache flush](http://horace0425.pixnet.net/blog/post/10184578-invalidate-flush-cache)
> ==Q:== write back vs write through
Cache 的兩個類型:
–Write Through
當寫數據進 Cache 時,也同時更新了相應的 Memory 裡的內容
–Write back
只是寫到 Cache 裡,Memory 的內容要等到 cache 保存的要被別的數據替換或者系統做 cache flush 時,才會被更新。
在 write-back cache 中,cache, memory 的資料通常是不同步的。
所以可能會多使用 dirty bit 來標示說 memory 的資料已經是舊的。
當 cache 要將資料清除時,得負責把資料寫回 memory ~
* reference: [cache invalidate VS cache flush](http://horace0425.pixnet.net/blog/post/10184578-invalidate-flush-cache)
* **指令介紹**
以下為 prefetch 的指令:
`void _mm_prefetch(char *p, int i)`
從地址 P 處預取長度為 cache line 大小的 data cache,參數 i 指示預取方式。
其中預取的方式分成 4 類:
* T0 預取資料到所有級別的快取
* T1 預取資料到第一級以外所有級別的快取
* T2 預取資料到第一、二級以外所有級別的快取
* NTA 預取資料到非臨時 cache 結構中,可以最小化對快取的污染
以下實驗 prefetch distance = 8,附上的數據為第一次執行結果
```
L1d cache: 32K
L1i cache: 32K
L2 cache: 256K
L3 cache: 3072K
```
* _MM_HINT_T0

```
naive: 443001 us
sse: 165594 us
sse prefetch: 99746.6 us
```
* _MM_HINT_T1

```
naive: 445146 us
sse: 167271 us
sse prefetch: 96087.9 us
```
* _MM_HINT_T2

```
naive: 446486 us
sse: 172104 us
sse prefetch: 89992.4 us
```
* _MM_HINT_NTA

```
naive: 375468 us
sse: 141636 us
sse prefetch: 122507 us
```
上面的四張圖,主要不同的部分是 sse prefetch 改動了 locality hint,可以觀察到的是 T0 的圖形 sse prefetch 抖動相當厲害,T1、T2的圖形就相對平順許多,NTA則是 sse prefetch 所需的平均執行時間相對其他三者來的高。
## prefetch distance
做這個實驗,第一個要考慮的是 distance 要設多少才合理,回憶一下公式:
$$ D \geq [\frac{l}{s}] $$
$l$ 是 prefetch latency
$s$ 是 the length of the shortest path through the loop body
參考 [carolc0708 HackMD 筆記](https://hackmd.io/s/B1lDZtO0#優化嘗試2-調整pfdist),嘗試算出 prefetch distance,但還不太清楚 mlc 工具如何使用。
* distance 1 ~ 20

* distance 1 ~ 100

* distance 1 ~ 20 (只有這個實驗每個距離重複做20次取平均)

可以看到 distance = 8 的時候效果最好
## 改變 array size
### 不同 array size 的實驗結果
從下圖可知,矩陣大小越大,時間差越多
> ==Q:== 不知道為何? 矩陣大小每次增加 96 時,和每次增加 64 時,所呈現的圖都是一高一低的齒狀圖形,尤其是 naive 和 sse 特別明顯。
> 測試過程中,沒有辦法從 4096 大小,執行到 4096+40*64 大小,中途電腦滑鼠會無法動,而所得的資料,會極其怪異,每次測試都不大相同。
>> 這是很棒的發現喔, 但是這樣的分佈是可以解釋的, 簡單回覆在下列文章
https://champyen.blogspot.tw/2017/05/cache-line.html
>> [name="champ"]
* 4096+i*64(i=0~29)

* 4096+(i)*64(i=30~44)

* 4096+(i)*64(i=45~59)

### 對上述關於不同 array size 的執行時間的分析
這是一個每次增加 64 的圖表分析(以此張圖作為分析)

這是一個每次增加 96 的圖表分析

在 i == 8 時,與上述 i == 12 時,是為同ㄧ大小 4864,並且能看出在 i == 8 時,與上述 i == 12 時的執行時間較久,表示 array size 在某些特定的值,執行時間較久。
* 實驗環境為 6MB cache 的 Core-i5 6300HQ 而且以 Intel Skylake 架構而言,是 64 bytes cache line 和 8-way associative cache (n way set associatve 表示為是指 n 個 cache line 為 1 個 set )。
* 由上述資訊可計算出具備6MB/64/8 = 12288 sets (entries)
* 這是一張 4 way set associative 的圖,1個 set 中有 4 個 cache line ,每個 cache line 為 64 bytes

>champ: 請注意 8-way 的意思, 另外針對文中的計算方式, 解釋一下 i == 4 的情況
#### **Cache line 填入次數計算**
發現不同 array size 的執行時間不同的原因,有部份是因為 conflict misses ,以下算法為計算發生衝突的次數,大於 8 表示 cache line 有相當高的機會早已被 replace 。
**首先考慮最簡單的 i == 0 的情況**
對於第 n 行的 cache line index 計算為: (X + 256*n) % 12288
1. 12288 / 256 = 48 (自 cache line 0 ~ 12288, 每次跳 256 可以消耗 48 行)
2. 12288 % 256 = 0 (每次的 cache line index shift)
所以填完 output 第一行來說, 這也代表著當資料(二維座標以(x,y)表示)自 (0, 0), (0, 1) .... (0, 4095) 要讀 (1, 0) 時
而**相關的 cache line 早已被反覆地填入了 4096/48 = 85.33次 > 8** (8-way associative)
當 CPU 需要讀入 (1, 0) 時, 因為讀入 (0, 0) 所讀入的 ++cache line 有相當高的機會早已被 replace++
**考慮 i == 1 的情況**
對於第 n 行的 cache line index 計算為: (X + 260*n) % 12288
1. 12288 / 260 = 47.26 ...
2. 12288 % 260 = 68
而260 與 68 兩者最小公倍數為 4420
4420/68 = 65 (表示反覆繞 cache line index 0 ~ 12288 到第 65 次才開始 cache line index 有所重疊)
而 4160/47.25 約為 88.02, 88.02 / 65 = 1.35 < 8
這表示在讀取 (1, 0) 時, 有很大的機會 (0, 0) 所填入的 cache line 還在
**考慮 i == 2 的情況**
於第 n 行的 cache line index 計算為: (X + 264*n) % 12288
1. 12288 / 264 = 46.55 ...
2. 12288 % 264 = 144
而 264 與 144 兩者最小公倍數為 1584
1584/144 = 11 (表示反覆繞 cache line index 0 ~ 12288 到第 11 次才開始 cache line index 有所重疊)
而 4224/46.55 約為 90.74, 90.74 / 11 = 8.25 > 8
**考慮 i == 4 的情況**
( 272 = 16*17 -> 4352*4 = 1024 * 17 )
於第 n 行的 cache line index 計算為: (X + 272*n) % 12288
1. 12288 / 272 = 45.17 ...
2. 12288 % 272 = 48
而 272 與 48 兩者最小公倍數為 816
816/48 = 17 (表示反覆繞 cache line index 0 ~ 12288 到第 17 次才開始 cache line index 有所重疊)
而 4352/45.17 約為 96.35, 96.34 / 17 = 5.66 < 8
**考慮 i == 8 的情況**
對於第 n 行的 cache line index 計算為: (X + 288*n) % 12288
1. 12288 / 288 = 42.6666....
2. 12288 % 288 = 192
而 288 與 192 兩者最小公倍數為 576
576/192 = 3 (表示反覆繞填 cache line index 0 ~ 12288 到第 3 次 cache line index 就有所重疊)
而 4608/42.666 約為 108, 108 / 3 = 36 > 8
這表示在讀取 (1, 0) 時, (0, 0) 所填入的 ++cache line 有相當高的機會早已被 replace++
**考慮 i == 12 的情況**
( 304 = 16*19 -> 4864*4 = 1024 * 19 )
於第 n 行的 cache line index 計算為: (X + 304*n) % 12288
1. 12288 / 304 = 40.42 ...
2. 12288 % 304 = 128
而 304 與 128 兩者最小公倍數為 2432
2432/128 = 19 (表示反覆繞 cache line index 0 ~ 12288 到第 19 次才開始 cache line index 有所重疊)
而 4864/40.42 約為 120.34, 120.34 / 19 = 6.33 < 8
#### **Perf 分析**
下面 perf 分析會使用到的 raw counter:
* r02D1 - L2 cache hit
* r10D1 - L2 cache miss
* r04D1 - L3 cache hit
* r20D1 - L3 cache miss
這幾個是屬於 Intel(R) Core(TM) i5-6300HQ CPU 的 raw counter
**矩陣寬度 4096 ( i == 0 )**
```
Performance counter stats for './naive_transpose' (5 runs):
52,244,018 cache-misses # 84.822 % of all cache refs ( +- 0.53% ) (60.11%)
61,592,825 cache-references ( +- 0.49% ) (70.33%)
24,347,389 L1-dcache-load-misses # 2.64% of all L1-dcache hits ( +- 0.54% ) (80.38%)
923,299,768 L1-dcache-loads ( +- 0.34% ) (70.69%)
252,005,875 L1-dcache-stores ( +- 0.38% ) (70.72%)
98,853 L1-icache-load-misses ( +- 1.44% ) (80.48%)
93,656 r02D1 ( +- 2.81% ) (80.49%)
16,115,520 r10D1 ( +- 0.53% ) (80.48%)
715,791 r04D1 ( +- 1.76% ) (49.09%)
15,077,830 r20D1 ( +- 0.38% ) (49.95%)
0.491992916 seconds time elapsed ( +- 0.25% )
```
**矩陣寬度 4160 ( i == 1 )**
```
Performance counter stats for './naive_transpose' (5 runs):
11,771,201 cache-misses # 18.490 % of all cache refs ( +- 0.97% ) (60.36%)
63,661,806 cache-references ( +- 0.64% ) (70.59%)
25,285,501 L1-dcache-load-misses # 2.62% of all L1-dcache hits ( +- 0.27% ) (80.46%)
966,458,779 L1-dcache-loads ( +- 0.30% ) (70.70%)
262,847,918 L1-dcache-stores ( +- 0.53% ) (70.70%)
90,397 L1-icache-load-misses ( +- 1.50% ) (80.46%)
71,785 r02D1 ( +- 5.09% ) (80.47%)
17,143,899 r10D1 ( +- 0.93% ) (80.49%)
14,871,389 r04D1 ( +- 3.39% ) (49.07%)
1,353,680 r20D1 ( +- 3.47% ) (50.15%)
0.327986759 seconds time elapsed ( +- 0.27% )
```
**矩陣寬度 4224 ( i == 2 )**
```
Performance counter stats for './naive_transpose' (5 runs):
16,372,167 cache-misses # 25.784 % of all cache refs ( +- 2.95% ) (59.63%)
63,497,659 cache-references ( +- 1.06% ) (69.75%)
25,652,130 L1-dcache-load-misses # 2.52% of all L1-dcache hits ( +- 0.39% ) (79.87%)
1,017,170,252 L1-dcache-loads ( +- 0.33% ) (69.91%)
269,196,901 L1-dcache-stores ( +- 0.61% ) (70.19%)
96,377 L1-icache-load-misses ( +- 5.27% ) (80.17%)
77,088 r02D1 ( +- 2.52% ) (80.21%)
17,741,750 r10D1 ( +- 1.13% ) (80.60%)
15,431,022 r04D1 ( +- 1.62% ) (50.21%)
2,636,131 r20D1 ( +- 6.35% ) (49.89%)
0.364426276 seconds time elapsed ( +- 2.39% )
```
**矩陣寬度 4288 ( i == 3 )**
```
Performance counter stats for './naive_transpose' (5 runs):
11,871,726 cache-misses # 18.148 % of all cache refs ( +- 0.59% ) (58.21%)
65,417,367 cache-references ( +- 1.04% ) (68.66%)
26,087,433 L1-dcache-load-misses # 2.50% of all L1-dcache hits ( +- 0.50% ) (79.10%)
1,043,204,339 L1-dcache-loads ( +- 0.36% ) (69.59%)
270,997,798 L1-dcache-stores ( +- 0.48% ) (71.24%)
85,889 L1-icache-load-misses ( +- 3.12% ) (81.26%)
75,277 r02D1 ( +- 8.37% ) (81.48%)
18,616,857 r10D1 ( +- 0.47% ) (81.50%)
17,770,666 r04D1 ( +- 1.43% ) (49.51%)
1,354,678 r20D1 ( +- 1.20% ) (48.34%)
0.345102593 seconds time elapsed ( +- 0.31% )
```
**矩陣寬度 4352 ( i == 4 )**
```
Performance counter stats for './naive_transpose' (5 runs):
27,367,328 cache-misses # 40.264 % of all cache refs ( +- 1.09% ) (60.22%)
67,969,453 cache-references ( +- 0.45% ) (70.36%)
27,607,107 L1-dcache-load-misses # 2.58% of all L1-dcache hits ( +- 0.21% ) (80.30%)
1,068,701,418 L1-dcache-loads ( +- 0.48% ) (70.47%)
285,498,785 L1-dcache-stores ( +- 0.21% ) (70.47%)
105,630 L1-icache-load-misses ( +- 3.80% ) (80.29%)
82,636 r02D1 ( +- 6.06% ) (80.32%)
18,488,940 r10D1 ( +- 0.25% ) (80.31%)
13,038,547 r04D1 ( +- 0.97% ) (49.47%)
6,317,678 r20D1 ( +- 1.24% ) (50.20%)
0.447266069 seconds time elapsed ( +- 0.15% )
```
**矩陣寬度 4416 ( i == 5 )**
```
Performance counter stats for './naive_transpose' (5 runs):
12,507,535 cache-misses # 17.064 % of all cache refs ( +- 1.09% ) (60.27%)
73,299,618 cache-references ( +- 0.57% ) (70.51%)
28,769,891 L1-dcache-load-misses # 2.65% of all L1-dcache hits ( +- 0.19% ) (80.44%)
1,085,556,969 L1-dcache-loads ( +- 0.31% ) (70.71%)
293,359,023 L1-dcache-stores ( +- 0.34% ) (70.70%)
103,116 L1-icache-load-misses ( +- 2.93% ) (80.45%)
94,335 r02D1 ( +- 2.75% ) (80.51%)
18,914,866 r10D1 ( +- 0.60% ) (80.50%)
18,532,942 r04D1 ( +- 1.29% ) (49.04%)
1,559,820 r20D1 ( +- 3.73% ) (50.15%)
0.368922933 seconds time elapsed ( +- 0.47% )
```
**矩陣寬度 4480 ( i == 6 )**
```
Performance counter stats for './naive_transpose' (5 runs):
17,432,548 cache-misses # 24.397 % of all cache refs ( +- 1.34% ) (59.57%)
71,453,677 cache-references ( +- 0.74% ) (69.69%)
29,038,043 L1-dcache-load-misses # 2.61% of all L1-dcache hits ( +- 0.60% ) (79.79%)
1,112,157,297 L1-dcache-loads ( +- 0.45% ) (69.69%)
302,607,996 L1-dcache-stores ( +- 0.18% ) (69.90%)
101,655 L1-icache-load-misses ( +- 2.72% ) (80.18%)
73,866 r02D1 ( +- 7.78% ) (80.41%)
19,485,295 r10D1 ( +- 1.02% ) (80.76%)
17,706,270 r04D1 ( +- 2.55% ) (50.13%)
2,713,080 r20D1 ( +- 4.95% ) (49.71%)
0.396332422 seconds time elapsed ( +- 0.69% )
```
**矩陣寬度 4544 ( i == 7 )**
```
Performance counter stats for './naive_transpose' (5 runs):
13,902,209 cache-misses # 18.656 % of all cache refs ( +- 1.75% ) (59.32%)
74,517,294 cache-references ( +- 0.37% ) (69.51%)
29,662,893 L1-dcache-load-misses # 2.60% of all L1-dcache hits ( +- 0.38% ) (79.67%)
1,141,767,135 L1-dcache-loads ( +- 0.30% ) (69.60%)
310,754,385 L1-dcache-stores ( +- 0.44% ) (70.27%)
100,052 L1-icache-load-misses ( +- 3.96% ) (80.69%)
78,525 r02D1 ( +- 11.19% ) (80.80%)
20,596,654 r10D1 ( +- 0.63% ) (81.03%)
18,682,574 r04D1 ( +- 1.42% ) (50.04%)
1,649,619 r20D1 ( +- 5.21% ) (49.40%)
0.393317067 seconds time elapsed ( +- 0.62% )
```
**矩陣寬度 4608( i == 8 )**
```
Performance counter stats for './naive_transpose' (5 runs):
52,088,899 cache-misses # 67.286 % of all cache refs ( +- 0.43% ) (60.18%)
77,413,791 cache-references ( +- 0.22% ) (70.31%)
30,787,255 L1-dcache-load-misses # 2.61% of all L1-dcache hits ( +- 0.24% ) (80.24%)
1,179,007,247 L1-dcache-loads ( +- 0.28% ) (70.36%)
322,404,442 L1-dcache-stores ( +- 0.11% ) (70.36%)
121,222 L1-icache-load-misses ( +- 3.05% ) (80.24%)
92,103 r02D1 ( +- 1.53% ) (80.24%)
20,832,962 r10D1 ( +- 0.03% ) (80.25%)
6,098,856 r04D1 ( +- 1.79% ) (49.53%)
14,443,071 r20D1 ( +- 0.37% ) (50.12%)
0.607956212 seconds time elapsed ( +- 0.16% )
```
**矩陣寬度 4672 ( i == 9 )**
```
Performance counter stats for './naive_transpose' (5 runs):
15,958,239 cache-misses # 20.419 % of all cache refs ( +- 3.27% ) (59.09%)
78,152,159 cache-references ( +- 1.10% ) (69.38%)
31,152,396 L1-dcache-load-misses # 2.55% of all L1-dcache hits ( +- 0.72% ) (79.63%)
1,222,813,455 L1-dcache-loads ( +- 0.51% ) (70.17%)
332,707,619 L1-dcache-stores ( +- 0.54% ) (70.60%)
106,836 L1-icache-load-misses ( +- 1.42% ) (80.44%)
84,821 r02D1 ( +- 9.55% ) (80.61%)
21,957,006 r10D1 ( +- 0.78% ) (80.98%)
20,497,673 r04D1 ( +- 2.06% ) (49.62%)
2,143,563 r20D1 ( +- 8.31% ) (48.80%)
0.425759875 seconds time elapsed ( +- 0.79% )
```
**矩陣寬度 4736 ( i == 10 )**
```
Performance counter stats for './naive_transpose' (5 runs):
20,448,149 cache-misses # 25.294 % of all cache refs ( +- 1.61% ) (60.18%)
80,841,857 cache-references ( +- 0.98% ) (70.37%)
32,879,456 L1-dcache-load-misses # 2.58% of all L1-dcache hits ( +- 0.52% ) (80.32%)
1,276,847,096 L1-dcache-loads ( +- 0.22% ) (70.53%)
341,388,116 L1-dcache-stores ( +- 0.20% ) (70.53%)
108,777 L1-icache-load-misses ( +- 2.26% ) (80.36%)
91,156 r02D1 ( +- 6.03% ) (80.36%)
22,110,026 r10D1 ( +- 0.67% ) (80.53%)
20,223,475 r04D1 ( +- 2.91% ) (49.23%)
3,025,741 r20D1 ( +- 7.02% ) (49.77%)
0.448701013 seconds time elapsed ( +- 0.74% )
```
**矩陣寬度 4800 ( i == 11 )**
```
Performance counter stats for './naive_transpose' (5 runs):
15,783,688 cache-misses # 18.399 % of all cache refs ( +- 0.77% ) (59.49%)
85,784,311 cache-references ( +- 0.59% ) (69.64%)
33,815,769 L1-dcache-load-misses # 2.59% of all L1-dcache hits ( +- 0.33% ) (79.75%)
1,308,067,594 L1-dcache-loads ( +- 0.26% ) (69.63%)
348,657,787 L1-dcache-stores ( +- 0.48% ) (69.63%)
107,826 L1-icache-load-misses ( +- 1.50% ) (79.75%)
78,116 r02D1 ( +- 12.74% ) (80.28%)
22,692,189 r10D1 ( +- 0.38% ) (81.01%)
21,970,177 r04D1 ( +- 2.12% ) (50.33%)
2,027,878 r20D1 ( +- 2.92% ) (49.71%)
0.435040386 seconds time elapsed ( +- 0.52% )
```
**矩陣寬度 4864 ( i == 12 )**
```
Performance counter stats for './naive_transpose' (5 runs):
36,841,281 cache-misses # 43.742 % of all cache refs ( +- 2.79% ) (59.33%)
84,224,880 cache-references ( +- 0.45% ) (69.55%)
34,265,504 L1-dcache-load-misses # 2.59% of all L1-dcache hits ( +- 0.22% ) (79.69%)
1,320,671,661 L1-dcache-loads ( +- 0.25% ) (69.93%)
352,445,476 L1-dcache-stores ( +- 0.39% ) (70.35%)
115,777 L1-icache-load-misses ( +- 1.93% ) (80.28%)
85,133 r02D1 ( +- 11.48% ) (80.54%)
23,172,324 r10D1 ( +- 0.16% ) (80.79%)
14,505,470 r04D1 ( +- 2.64% ) (49.79%)
8,711,029 r20D1 ( +- 4.49% ) (49.35%)
0.567957430 seconds time elapsed ( +- 1.21% )
```
### Column major 與 Row major
* 分析 array size 與 總執行時間
```clike=
int repeat_times = 1000000000;
long array[array_size];
for (int i = 0; i<array_size; i++)
array[i] = 0;
int k = 0;
while (int j++ < repeat_times){
if (k == array_size)
k=0;
c = array[k++];
}
```

* 從上圖可以發現從 16*4 bytes 後的數值增加幅度很大,原因是當 array sizes 小於 64Bytes 時,array 可能落在同ㄧ條 cache line 中。然而一個元素的存取就會使整條 cache line 被填充,也就是說當我接下來要存取的元素,不在同一個 cache line 的話,就會使另一條 cache line 被填充。而后面的若干個元素則會受益於 cache 带来的加速。所以當數组大於 64 Bytes 时,一定需要两條 cache line,而由於 cache line 填充的时間遠高於數據在同一個 cache line 存取的時間,因此多一次快取填充,會使總時間增大。
* 下圖陣列存取的方式,第一種會快於第二種,因為
在以 row major 存取陣列時,當你第一次讀取,會讀取 a[0][0] 的記憶體地址,此時,整個 cache line 都被從主記憶體取到 cache 中,並且,接著讀取同一個 cache line 中的其他數值會非常快,所以接著存取 a[0][1] ~ a[0][7] 會很快。
但在以 col major 存取陣列時,當你第一次讀取,會讀取 a[0][0] 的記憶體地址,此時,整個 cache line 都被從主記憶體取到 cache 中,然而,接著存取到的卻是 a[1][0] ,因為不在同一個 cache line 中,所以會使另一條 cache line 被填充,導致時間花費久。
原文網址:https://read01.com/D5NaOa.html

在不同 array size 中,可以從下圖看出 row major 比 col major 的執行時間快
* **row major**

* **col major**
